Panel
Frontier in Multimedia
Time: 2019/12/18 10:30~12:00, Location: Auditorium
Session Chair: Liqiang Nie
In this panel, we have invited four leading scholars in our multimedia community to introduce recent advances in multimedia research, including compression, visual recognition, domain adaptation and differentiable neural architecture search (NAS).
Invited Panelists and Talks:
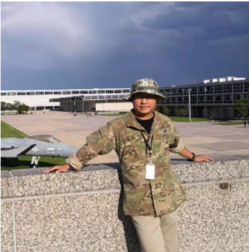
Title: “Recent Advances in Deep Learning for Compression” by Zhu Li, University of Missouri, USA
Abstract: Deep learning has found many applications in vision and image processing tasks with impressive results. In recent years deep learning based tools are also getting into the compression and have demonstrated quite some new performance gains in improving the standard based compression technology like HEVC, and at the same time, purely learning based and data driven compression also starts showing promises. I will review some current state of the art in the area, and discuss the new opportunities, challenges, and directions.
Bio: Prof. Zhu Li. Zhu Li is now an Associate Professor with the Dept of Computer Science & Electrical Engnieering (CSEE), University of Missouri,Kansas City, and the director of the new NSF Center for Big Learning at UMKC . He was an AFRL summer visiting professor at the US Air Force Academy , Colorado Springs, Summer of 2016,'17,'18. He received his PhD in Electrical & Computer Engineering from Northwestern University, Evanston in 2004. He was Sr. Staff Researcher/Sr. Manager with with Samsung Research America's Multimedia Standards Research Lab in Richardson, TX, 2012-2015, Sr. Staff Researcher/Media Analytics Group Lead with FutureWei (Huawei) Technology's Media Lab in Bridgewater, NJ, 2010~2012, and an Assistant Professor with the Dept of Computing, The Hong Kong Polytechnic University from 2008 to 2010, and a Principal Staff Research Engineer with the Multimedia Research Lab (MRL), Motorola Labs, from 2000 to 2008. His research interests include image/video, light field and point cloud compression, deep learning-based compression schemes, image/video denoising and super-resolution, video hashing and identification, as well as the video communication system issues like joint source-channel coding, rate-distortion optimization and communication resource management. He has 46 issued or pending patents, 100+ publications in book chapters, journals, conference proceedings and standard contributions in these areas. He is an IEEE senior member, associated editor (2020~) for IEEE Trans on Image Processing , associated editor (2015~19) for IEEE Trans.on Multimedia, and associated editor (2016~19) for IEEE Trans on Circuits & System for Video Technology, associated editor (2015~18) for Journal of Signal Processing Systems (Springer), steering committee memeber of IEEE ICME, elected member (2014-2017) of the IEEE Multimedia Signal Processing (MMSP) Tech Committee, elected Vice Chair (2008-2010), Standards Liaison (2014-2016) of the IEEE Multimedia Communication Technical Committee (MMTC), member of the Best Paper Award Committee, ICME 2010, co-editor for the Springer-Verlag book on "Intelligent Video Communication: Techniques and Applications", and " Multimedia Analysis, Computing and Communication,". He received the Best Poster Paper Award at IEEE Int'l Conf on Multimedia & Expo (ICME), Toronto, 2006, and the Best Paper (DoCoMo Labs Innovative Paper) Award at IEEE Int'l Conf on Image Processing (ICIP), San Antonio, 2007.
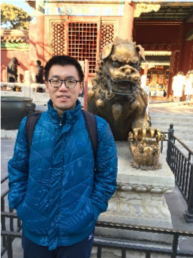
Title: “Differentiable Neural Architecture Search: Promises, Challenges, and Our Solutions” by Lingxi Xie, Noah's Ark Lab, Huawei Inc, China
Abstract: Recently, differentiable neural architecture search has attracted a lot of research attention, mainly due to its high efficiency in exploring a large search space. However, researchers also noticed significant drawbacks in its instability, which obstacles us from applying it freely to a wide range of vision tasks. In this talk, we first explain why differentiable search is important and promising, and then we delve deep into the reason for instability, based on which we present our solutions for alleviating it. We hope that our research can inspire future work in this direction.
Bio: Dr. Lingxi Xie. Lingxi Xie is currently a senior researcher at Noah's Ark Lab, Huawei Inc. He obtained B.E. and Ph.D. in engineering, both from Tsinghua University, in 2010 and 2015, respectively. He also served as a post-doctoral researcher at the CCVL lab from 2015 to 2019, having moved from the University of California, Los Angeles to the Johns Hopkins University. Lingxi's research interests lie in computer vision, in particular, the application of deep learning models. His research covers image classification, object detection, semantic segmentation, and other vision tasks. He is also interested in medical image analysis, especially object segmentation in CT or MRI scans. Lingxi has published over 40 papers in top-tier international conferences and journals. In 2015, he received an outstanding Ph.D. thesis award from Tsinghua University. He is also the winner of the best paper award at ICMR 2015.
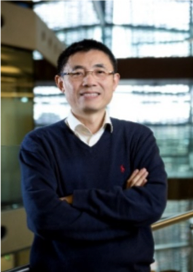
Title: “Webly Supervised Fine-Grained Visual Recognition” by Jian Zhang, University of Technology Sydney, Australia
Abstract: Labeling objects at the subordinate level typically requires expert knowledge, which is not always available from a random annotator. Accordingly, learning directly from web images for fine-grained visual classification (FGVC) has attracted broad attention. However, the existence of noise in web images is a huge obstacle for training robust deep neural networks. To this end, we propose a novel approach to remove irrelevant samples from the real-world web images during training, and only utilize useful images for updating the networks. Thus, our network can alleviate the harmful effects caused by irrelevant noisy web images to achieve better performance.
Bio: Prof. Jian Zhang. Dr. Jian Zhang received the Ph.D. degree in electrical engineering from the University of New South Wales (UNSW), Sydney, Australia, in 1999. From 1997 to 2003, he was with the Visual Information Processing Laboratory, Motorola Labs, Sydney, as a Senior Research Engineer, and later became a Principal Research Engineer and a Foundation Manager with the Visual Communications Research Team. From 2004 to July 2011, he was a Principal Researcher and a Project Leader with Data61/NICTA, Sydney. He is currently an Associate Professor and Director of Multimedia Data Analytics Lab with the Global Big Data Technologies Centre, School of Electrical & Data Engineering, Faculty of engineering and Information Technology, University of Technology Sydney, Sydney. Prof Zhang’s research interests include multimedia signal processing, computer vision, pattern recognition, visual information mining, human-computer interaction and intelligent video surveillance systems. Prof Zhang has co-authored more than 150 paper publications, book chapters, patents and technical reports from his research output, he was the co-author of eight granted US and China patents. Dr. Zhang is an IEEE Senior Member and Associated Editor, IEEE Transactions on Multimedia. He was Associated Editor, IEEE Transactions on Circuits and Systems for Video Technology (T-CSVT) from 2006 – 2015, He chaired a number of IEEE International conferences including Technical Program Chair of 2008 IEEE Multimedia Signal Processing Workshop, Leading General Co-Chair, of 2012 International Conference on Multimedia and Expo (ICME) in Melbourne Australia, of 2019 Technical Program Co-Chair of 2014 IEEE International Conference on Visual Communications and Image Processing, Technical Program Co-Chair of IEEE ICME 2020 in London and Leading General Chair of 2019 IEEE International Conference on Visual Communications and Image Processing in Sydney Australia.
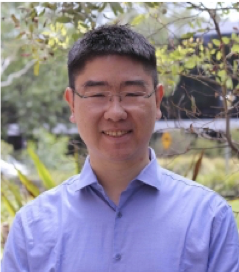
Title: “Content-level Domain Adaptation” by Liang Zheng, Australian National University, Australia
Abstract: Domain adaptation (DA) has been an important research problem, aiming to reduce the impact of domain gaps. Given images in the source and target domains, existing DA methods typically perform domain alignment on the feature- or pixel-level. In this talk, I will introduce a new scheme named content-level domain adaptation, where the source domain consists of images simulated by 3D renderers like Unity, and the target domain contains real-world images. Different from existing DA methods, content-level DA is featured by an editable source domain, where the source images can be freely edited in Unity in terms of illumination, viewpoint, background, etc. Using a supervision signal from the target domain, we design an attribute descent method to automatically generate a source domain that aligns well with the distributions in the target domain. On several vehicle re-identification datasets, we show that our method effectively edits the content of the source domain, generates consistent source images with the target domain, and brings about consistent improvement on top of traditional DA methods.
Bio: Prof. Liang Zheng. Dr Liang Zheng is a Lecturer, CS Futures Fellow and ARC DECRA Fellow in the Research School of Computer Science in the Australian National University. He obtained both his B.S degree (2010) and Ph.D degree (2015) from Tsinghua University. He has published over 40 papers in highly selected venues such as TPAMI, IJCV, CVPR, ECCV, and ICCV. He makes early attempts in large-scale person re-identification, and his works are positively received by the community. Dr Zheng received the Outstanding PhD Thesis and the Wen-Tsun Wu Award from Chinese Association of Artificial Intelligence and DECRA award from the Australian Research Council. His research has been featured by the MIT Technical Review, and four papers are selected into the computer science courses in Stanford University and the University of Texas at Austin. He serves as an Area Chair/Senior PC/Session Chair in ECCV 2020, AAAI 2020, IJCAI 2020, IJCAI 2019, 2020 ICPR 2018, ICME 2019, and ICMR 2019, and organized tutorials and workshops at ICPR 2018, ECCV 2018, CVPR 2019 and CVPR 2020.